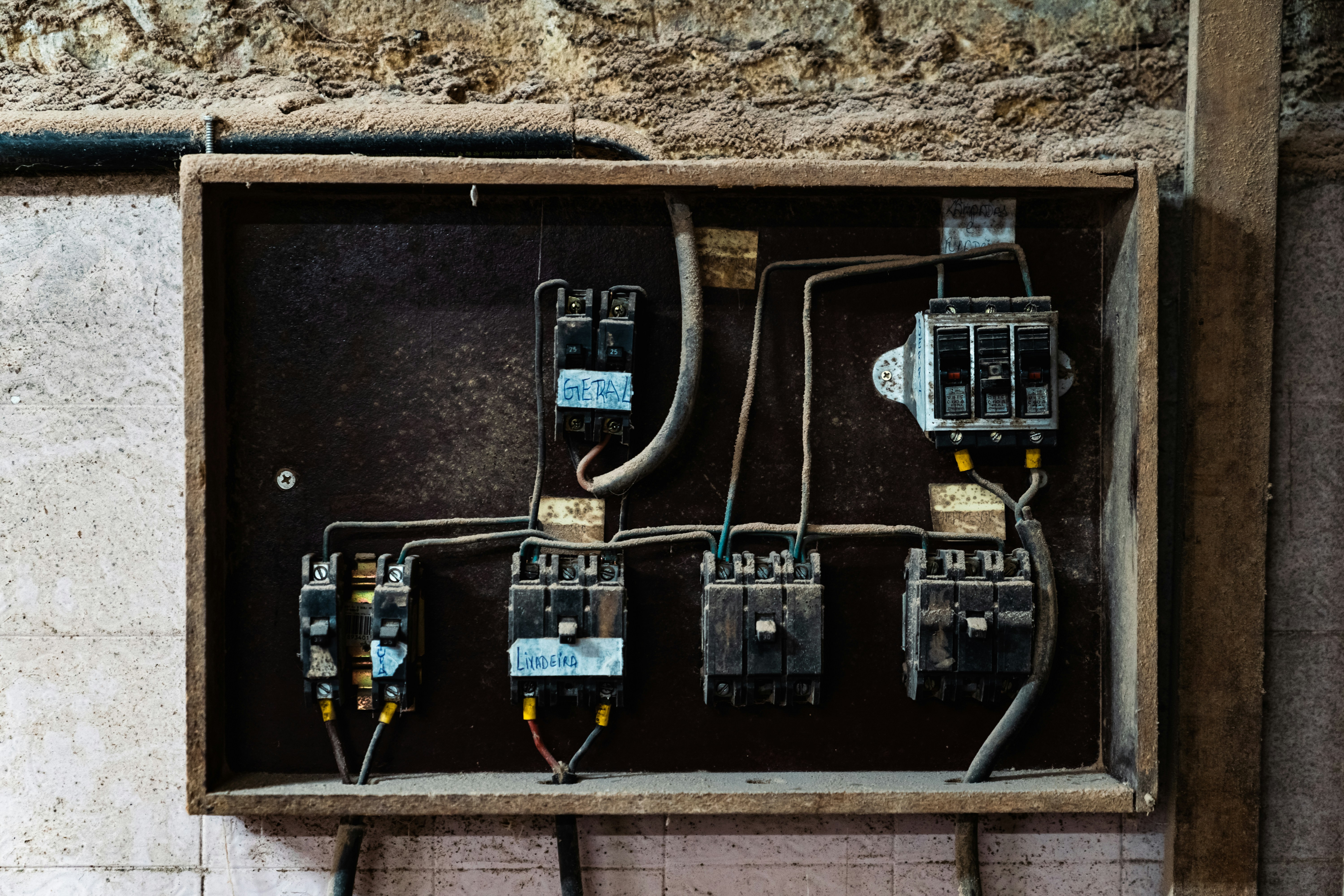
Multi-load metering for reduced costs, accurate billing & tailored tariffs
Ever wondered how mini-grid operators can reduce costs, accurately bill customers, and offer smarter, tailored tariffs—all at once?
CrossBoundary’s Innovation Lab, funded with UK aid from the UK government via the Transforming Energy Access platform, is evaluating the pioneering use of multi-load smart meters equipped with Non-Intrusive Load Monitoring (NILM) technology. This approach enables operators to disaggregate energy usage from a single meter, accurately identifying individual appliance consumption without needing additional, costly hardware.
In traditional mini-grid setups, operators install separate meters to gain detailed insights into individual household consumption. This can be done using a normal smart meter dedicated to the load, or sometimes installing IoT devices into appliances, a process known as intrusive load monitoring. Standard meters can only track overall household energy usage without distinguishing between appliances. Multiple meters become necessary if differentiated usage across appliances is required, which significantly raises costs for both hardware and ongoing service charges and limits scalability.
But why would a developer want to monitor disaggregated loads?
- To implement differentiated tariffs: By understanding how and when different appliances consume energy, operators can create targeted “type of use” and “time of use” tariffs. For instance, productive uses, such as milling or refrigeration, might be priced differently from household uses, such as clean cooking, thereby incentivizing efficient energy utilization. Differentiated tariffs and tailored offerings can drive increased consumption and revenue by encouraging productive energy use during off-peak periods or specific appliance categories.
- To optimize grid operations: Detailed insights enable operators to balance loads, enhance system efficiency, and prolong equipment lifespan by mitigating strain during peak hours.
- To enhance customer engagement and satisfaction: With granular data, operators can offer personalized recommendations to customers on energy savings, usage patterns, and efficient appliance utilization, increasing customer satisfaction and loyalty.
- For carbon credits: By understanding exactly when appliances such as EPC cookers are being used, developers can offer low-cost electricity and claim carbon credits to lower the cost of acquisition and/or increase revenues.
- To enable predictive maintenance: Continuous monitoring of appliances can help developers identify anomalies or changes in electrical data. The developer can then suggest replacing certain products – potentially through developer-sourced financing.
How does Non-Intrusive Load Monitoring (NILM) technology work?
PowerPay Africa has developed an algorithm to identify patterns associated with individual appliances by analyzing electrical data from a single meter. Using this AI model, granular insights into household or SME energy consumption are broken down into individual appliance components by applying advanced Machine Learning techniques. The steps in designing this algorithm were:
Distinguishing between signals:
The plots in Figure 1 show the characteristics of two different appliances over time. We’re evaluating an EPC Cooker against a Freezer to showcase three signals. To analyze these patterns, PowerPay created a labelled training dataset in the lab and trained a CNN-LSTM model, a type of deep learning architecture that combines convolutional layers for capturing signal transitions with Long Short-Term Memory (LSTM) that excels at learning temporal patterns over time.
Figure 1: Power, current, and voltage for an electric pressure cooker and freezer

In this example, the training data contains time-based signals, like power usage, voltage, and current, which change as different appliances are turned on or off. The Electric Pressure Cooker has a clear “pulse” pattern, while the Freezer cycles in short bursts and draws less current. The model can learn such differences.
To detect appliances, the LSTM model learns how electrical signals behave over time for specific appliances and their combinations:
- From lab data to pattern recognition: The LSTM was trained using two-second electrical data samples from the PowerPay lab. By analyzing these samples across various appliance combinations, the model developed the ability to recognize each appliance’s unique “signature” in the electrical signals.
- From patterns to real-time detection: With training complete, the CNN-LSTM model now processes new electrical data streams and identifies active appliances by matching observed patterns against its learned signatures. Our innovation journey continues as we prepare to validate this model’s performance in actual field conditions.
The CNN layers act like a sharp-eyed observer noticing every little signal change, while the LSTM acts like a detective, examining how the signals change over time and using its memory of past patterns to determine which appliances are responsible for those changes.
Figure 2: Time series of energy consumption disaggregated by appliance type
Figure 2 showcases consumption over time for various appliances, demonstrating how the algorithm effectively disaggregates energy consumption by appliance type throughout the day. It provides valuable, appliance-level insights from a single metering point by identifying distinct appliance consumption patterns. Examples include electric mills, pressure cookers, freezers, and other devices. Producing granular disaggregated data enables operators to implement targeted tariffs, optimize operational strategies, and encourage efficient energy use.
Anticipated results:
Our key hypotheses and anticipated results for this study include:
- Using a single multi-load meter instead of multiple meters will reduce the metering cost per connection by at least 20%
- Using locally manufactured multi-load meters will reduce the metering cost per connection by 10% compared to commercially available meters
- Implementing “type of use” and “time of use” tariffs through PowerPay’s multi-load smart meters combined with the AI model will increase consumer energy consumption by 20% and revenue by 10%
- “Time of use” tariffs will boost daytime utilization by 10%
- The multi-channel meter will accurately distinguish at least three appliance frequencies simultaneously
- Differentiated tariffs for Productive Use of Energy (PUE) will increase utilization rates by 10% and boost specific appliance usage by 25%
We anticipate that the results from this study will also be used to inform the application of AI modelling in driving energy efficiency and minimizing energy wastage through smart home and SME level data analytics that can allow electricity consumers to make decisions around appliance utilization and energy management. There is an opportunity to pioneer the use of AI in carbon digital Monitoring, Reporting and Verification (dMRV), particularly where smart meters are available, thus reducing the cost of deploying an additional layer of intrusive load monitoring technology.
Preliminary results:
PowerPay’s algorithm achieved an F1 score of 89% across appliances. This means it could accurately identify appliance usage in most cases while keeping false alarms low—a promising sign of reliability.
When applied to a customer’s energy data, the model identified several extended usage sessions aligned with typical electric pressure cooker (EPC) cooking cycles. This early result suggests the model’s ability to recognize real appliance behavior, even without ground-truth labels.
While there’s still more validation work ahead, especially in live field settings, these early results are encouraging signs that the model can generalize well beyond the lab, an important step as we move toward broader deployment.
Stay tuned as we roll out this groundbreaking study in Kenya, and please get in touch if you have additional data that you’d like us to include in our publications in the future!